Accelerating the Future of Life Sciences with AI: Empowering Science and Process
November 26, 2024
Art Morales, Ph.D
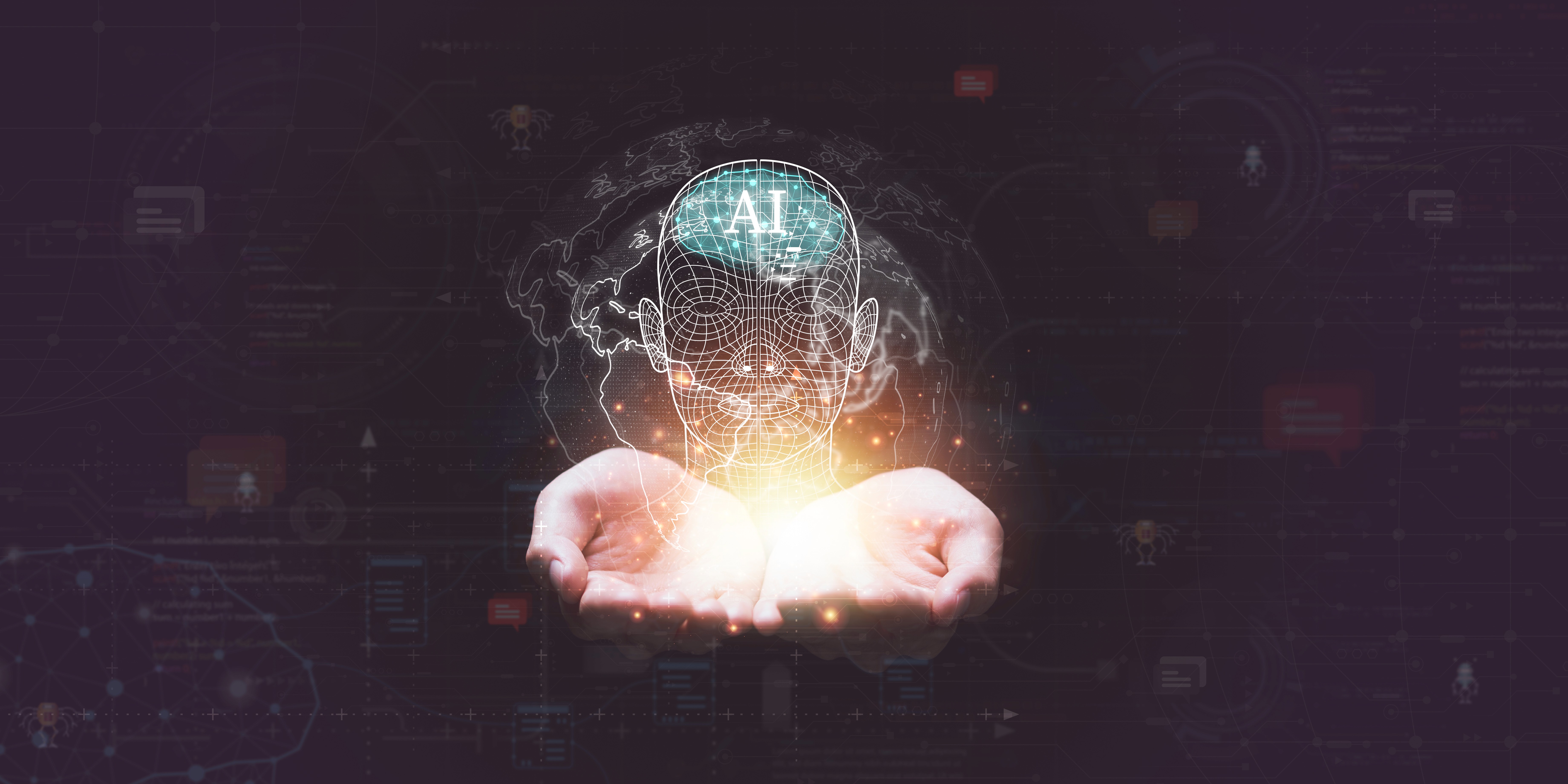
I was recently part of an awesome panel discussing “The future of AI in life sciences” (sponsored by the Greater Boston chapter of Women in Bio) where we discussed several interesting applications of AI in Life Sciences in addition to the changing workforce and the opportunity for women to advance in the field. While preparing for it, I started to put some thoughts together and wanted to complement and amplify some of the themes discussed there.
AI’s role in life sciences is reshaping the field across two major areas: driving scientific discovery and optimizing research processes. From decoding molecular structures to rethinking laboratory workflows, AI offers powerful tools to address complex scientific problems.
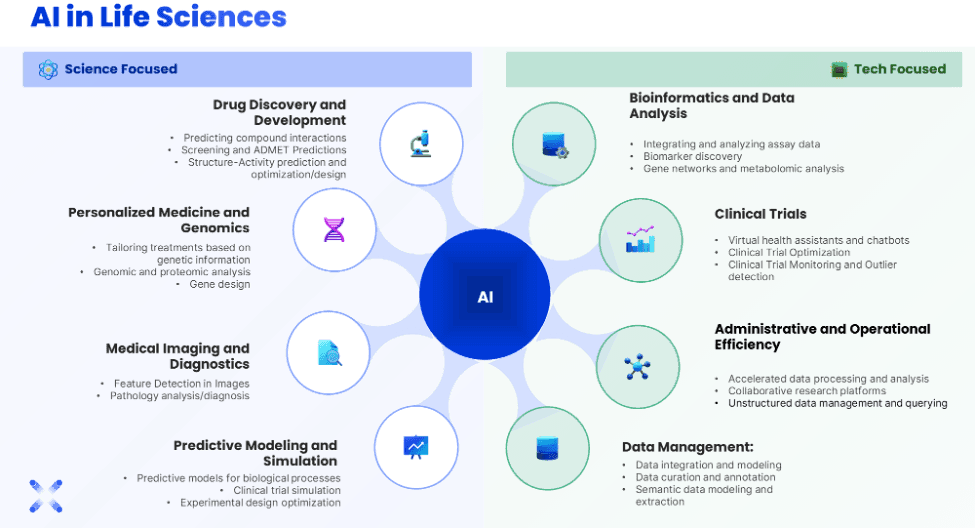
Figure 1: Examples of AI Applications in Life Sciences and how AI can help. On the right side, AI generally helps by providing technology that improves processes and productivity, while the applications on the left side are usually focusedon using AI to answer scientific questions.mWhile there’s often overlap between the areas, this diagram helps bring order to the landscape.
AI as an Enabler, not a Replacement: Transforming the Role of the Scientist
A frequent misconception is that AI may replace scientists in life sciences. In reality, AI’s value lies in augmenting and expanding the scientist’s capabilities. AI helps researchers to explore beyond the limits of traditional trial-and-error and conventional experimentation, enabling a more expansive, exploratory approach. Rather than restricting human expertise, AI amplifies it, allowing researchers to simulate and test conditions far beyond manual reach. For instance, AI models can propose a wide array of potential pathways or biological processes that scientists can refine with their judgment, enhancing both depth and speed of discovery. As a trained molecular/structural biologist, I learned to think about genetic-based screens in bacteria, letting life try out as many combinations as possible in a culture dish. This led to me always wanting to “Boil the ocean” and getting into statistical arguments like the multiple-observation problems for significance. Still, a well-designed ocean boil can save time and get more comprehensive results. AI approaches can overcome many of the limitations of scope as the limits are more about resources and cost rather than conceptual and those can be managed.
With this shift, researchers transition from designing every individual experiment to interpreting AI-driven insights, accelerating the pace of scientific exploration. I can’t wait to go back to my statistical sparring partners and show them the power of thinking beyond a teacup boil.
AI in Discovery: Deciphering the Language of Life
One of AI’s most profound contributions to life sciences lies in enhancing our understanding of complex biological systems. Large-scale sequence models, for example, are helping to decode proteins and RNA structures by analyzing sequences in ways that traditional methods couldn’t achieve. Much like language models in NLP, these models capture the “language” of molecular biology, finding patterns, structures, and possible functions. Although some optimization challenges remain, these AI models have reached a level where they’re invaluable in foundational research, aiding scientists in hypothesizing new functions and identifying relationships within massive datasets. Through this, researchers can uncover insights into protein functionality, molecular interactions, and even genetic markers for diseases.
One area that I’m very excited about is where GenAI agents can serve to bridge the gap across scientific domains. It has often been difficult for a chemist to independently analyze and interpret results from biological assays in context, understanding their limitations and potential. The same is also true for biologists trying to triage compounds based on their manufacturing complexity/etc. All these data are in the lab notebooks, but it’s sometimes hard to decode and interpret them across domains. Imagine if those notebooks were properly annotated with enterprise semantics in a way that allowed GenAI agents to translate and contextualize information across domain areas. In other words, a biologist interested in chemistry or CMC results would be able to get not just the results they needed but also see them translated into the appropriate level of detail and context for them to interpret them.
AI in Research: Enabling a New Kind of Lab Assistant
AI’s impact extends beyond scientific insight to transforming laboratory operations and processes. Generative AI, for instance, can act as a “virtual lab assistant,” handling tasks like literature searches, summarizing findings, and drafting experiment designs. Imagine a researcher discussing an experiment idea and an AI assistant promptly suggesting relevant studies, methodologies, or biomarkers to consider. Though not flawless, this assistance frees up researchers to focus on interpreting data and forming hypotheses, expediting the research process without sacrificing quality. Paired with domain knowledge, AI complements human expertise, creating an environment where innovation thrives.
AI in Clinical Development: Giving Drugs the Best Chance to Show Their Effectiveness
Ask any layperson as to why drugs cost so much, and they will say that it’s because of greed. Ask someone in Pharma and they’ll tell you that the process is long and complicated, and we fail often. The drugs that make it through need to recoup the cost of all those failures.
Taken at face value, a clinical trial should objectively test if a drug works and if it is safe. But the interesting thing is that every drug that fails in a Phase III trial, has had a positive signal in a Phase II trial. Granted, drugs can fail because of toxicity and side effects and sometimes we don’t see those issues until we get enough patients… But why do we see drugs show poor efficacy in a Phase III trial when the readout in Phase II was positive? Surely, the drug didn’t change (except for some exceptions)… so what did? Quite often the thing that changed is that the trial got more complex. Phase III trials usually involve more patients, more countries, and more clinical sites. Each site is basically running an independent version of the trial and we hope that the statistical power is good enough to show efficacy or superiority over the control arm. Unfortunately, trials often fail because of how they were executed and not because of the drug.
Clinical trial monitoring is a huge cost in every trial, and we spend a lot of time reviewing what happens at each site to identify issues that could be corrected in flight. But this is a hard problem to solve. We monitor as many KPIs (Key Performance Indicators) as we can, but they don’t tell the whole story. To supplement them, reports are regularly written about each site based on site visits by clinical monitors, and then hopefully someone reviews them. Unfortunately, the reports are then locked in a folder or database and only reviewed when there’s a specific reason to (like a regulatory audit or an obvious issue in the trial).
GenAI is perfectly suited to analyze unstructured data such as these reports. What if we had a system that consistently analyzed those reports to identify potential issues or blind spots at sites (or region or country level) and supplement the traditional monitoring efforts? The good news is that we can. The better news is that we know it can help. This is a perfect application of GenAI and something we should embrace. In the end, imagine if through better monitoring of those reports we could identify behaviors at sites that potentially increased placebo response and we could address them right away…
AI as a Knowledge Bridge: From Data to Insight
AI serves as an essential bridge from raw data to meaningful insight. Traditionally, moving from data collection to analysis has been time-intensive, requiring detailed processing and literature review. With generative AI, researchers can quickly access and analyze extensive knowledge bases, asking questions and receiving insights in minutes rather than hours. This reduces the gap from data to hypothesis generation, helping scientists identify patterns and contextually enrich their findings with related experimental data or theoretical insights. It also empowers researchers to rely less on others to ask questions, a savvy scientist can use GenAI to develop code that they can run to analyze their data faster and combine it with external results in hours instead of having to assemble a team of engineers and computational scientists to build the tool over a few weeks.
Embracing Tangents as part of the discovery process
A unique advantage of generative AI is its ability to facilitate unexpected exploration. In scientific research, pursuing a tangent can sometimes reveal valuable insights unrelated to the original hypothesis. AI can encourage this serendipitous process by surfacing novel connections that researchers may not have considered, sparking new questions or lines of inquiry. For example, an AI tool analyzing a specific protein might reveal relevant, unexpected pathways or interactions, potentially leading to breakthroughs in understanding biological processes.
In the end, AI is reshaping life sciences not by replacing scientists but by extending their reach and refining their methods. By enabling faster hypothesis generation, reducing manual tasks, and encouraging exploration, AI helps researchers make connections that might otherwise go unnoticed. As life sciences continue to integrate AI-driven insights, scientists gain the freedom to focus on critical analysis and higher-level decision-making. In this evolving partnership, AI doesn’t diminish human expertise—it elevates it, opening doors to discoveries that might have been unattainable in the past. Domain expertise is still paramount as is the ability to reason and ask questions. But we shouldn’t think that asking questions is just “prompt engineering”. The role of the scientist is and will remain to be curious, to ask the “what if” and to use the tools available to seek those answers. AI and GenAI accelerate the process, and I can’t wait to see what’s next!