The Jury is In! … implementing FAIR Data Principles Delivers Value in Pharmaceutical R&D
November 12, 2024
John Apathy, Tom Plasterer, Ph.D.
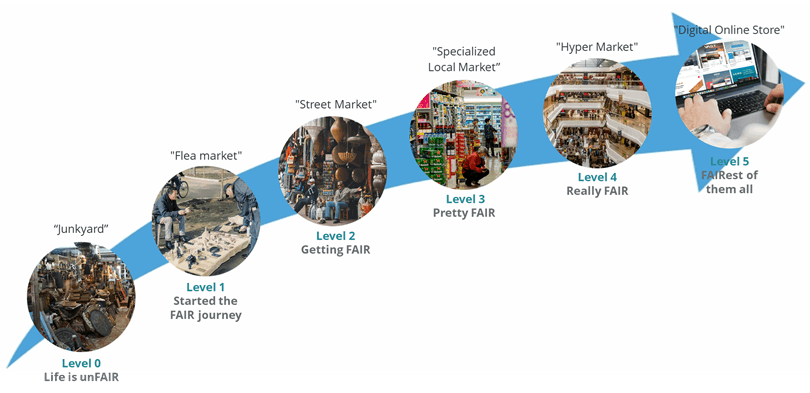
The pharmaceutical industry has long been grappling with the complexity of managing vast amounts of data and content generated from research experiments, genomic analysis, clinical trials, and regulatory submissions. This data, a goldmine of insights that has become increasingly the focus of AI, Machine Learning and advanced analytics, often remains untapped due to its sheer volume, variety, and velocity — and the underinvestment in clean, well-described and well-managed data.
However, the adoption of FAIR data principles — Findable, Accessible, Interoperable, and Reusable — has emerged as an important North Star, guiding organizations towards unlocking the true potential of their data assets. Value from FAIR data takes a variety of forms, but generally fits the following value framework for improvements in Cost, Speed, Effectiveness, and Trust.
A Business Value Framework for FAIR Data
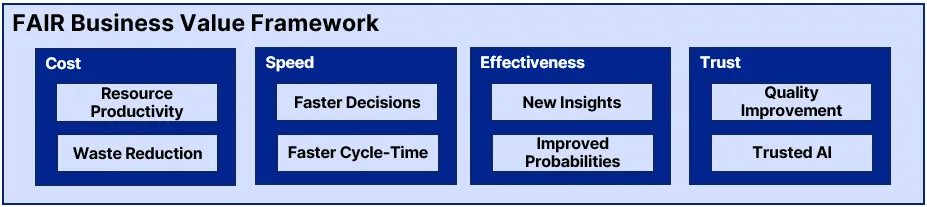
From learned experience, not everything within this framework fits seamlessly into a quantitative argument when making the case to investment in FAIR data. Often, an organization must rely on building a solid qualitative case, relying on prior examples of value from case studies or other sources to build the value story. Expressing the business value story is perhaps the most important success factor for moving from awareness to engagement to support across the change management journey required to drive sustained interest and sponsorship of a FAIR data transformational journey.
The FAIR Advantage: A Catalyst for Innovation
The pharmaceutical industry, with its relentless pursuit of life-saving therapies, stands to benefit significantly from the implementation of FAIR data principles. By making data more findable, accessible, interoperable, and reusable, organizations can accelerate drug discovery, optimize clinical trials, and improve patient outcomes.
Example sources of business value
Accelerated Drug Discovery and Development:
Efficient Data Retrieval: FAIR data implementations enable researchers to quickly and easily locate relevant data, reducing time spent on data searching and preparation.
Enhanced Data Analysis: By standardizing data formats and improving data quality driving deeper, more trustworthy data, researchers can conduct more sophisticated analyses, leading to faster insights and breakthroughs.
Facilitated Collaboration: FAIR data promotes seamless collaboration between scientists, researchers, and clinicians, fostering knowledge sharing and accelerating innovation.
2. Optimized Clinical Trials to Speed the Pipeline:
Improved Patient Recruitment: FAIR data implemented across patient data sources can help identify eligible patients more efficiently, leading to faster patient recruitment and trial initiation.
Enhanced Data Governance: Organizations can reduce the risk of data errors and avoid costly trial delays through improved data stewardship and governance by implementing FAIR data principles.
Streamlined Data Analysis: FAIR data facilitates data analysis and reporting, enabling researchers to draw meaningful conclusions from large and complex datasets.
3. Enhanced Regulatory Compliance:
Simplified Data Submission: FAIR data implementations can help organizations meet regulatory requirements by ensuring that data is well-organized, accessible, and compliant with industry standards.
Reduced Risk of Audit Failures: By implementing robust data management practices, organizations can minimize the risk of audit findings.
Accelerated Regulatory Approval: Efficient data access and analysis can expedite regulatory review processes, leading to faster drug approvals and market entry.
Trusted AI: FAIR data can deliver a higher-level of assurance for reproducibility and trust in the application of safe, trusted AI capabilities.
4. Improved Decision Making:
Data-Driven Insights: FAIR data enables data-driven decision making by providing access to accurate, reliable, and timely information.
Reduced Bias and Increased Objectivity: By standardizing data and analysis processes, organizations can reduce the impact of human bias and improve the objectivity of decision-making.
Enhanced Risk Management: FAIR data can help identify and mitigate risks early on, leading to more effective risk management strategies.
Real-World Examples of FAIR Data in Action
Several pharmaceutical companies have adopted a data-centric mindset, committing themselves to maturing their data capabilities by embracing FAIR data principles. These companies are reaping the rewards of this investment in people, process, technology, and data. For instance, some organizations have implemented new data strategies for modernizing their data architectures. This has involved implementation of data platforms, semantic capabilities, and moving toward a data product-centric and data marketplace capability. Others have invested in data governance and data quality initiatives to ensure data accuracy and consistency.
Overcoming Challenges and Ensuring Successful Implementation
While the benefits of FAIR data are clear, implementing FAIR principles is transformational and can be challenging. Some common hurdles include:
Data Silos: Breaking down data silos and promoting data sharing can be difficult, especially in large, decentralized organizations.
Data Quality Issues: Poor data quality can hinder the effectiveness of FAIR data initiatives.
Lack of Technical Expertise: Organizations may lack the technical expertise to implement and maintain FAIR data systems.
Resistance to Change: Change management can be challenging, as employees may be resistant to adopting new data practices.
Based upon our own personal experience and through the practical and diverse experiences at XponentL Data, we have helped clients to overcome these challenges. Common strategies include:
Establish a Clear Data Strategy: Define a clear data strategy that aligns with the organization’s overall business objectives.
Build-out the organization’s Semantic Capabilities: Design the technology stack that will build and maintain the organization’s ability to describe and maintain its “language for data” — e.g., ontologies, taxonomies, controlled vocabularies, master and reference data.
Invest in Data Governance: Implement robust data governance practices to ensure data quality, security, and compliance.
Build a Strong Data Culture: Foster a data-driven culture that encourages and incentivizes data sharing, collaboration, and innovation.
Leverage Technology: Utilize advanced technologies such as modern data platforms, semantic capabilities, knowledge graphs, and AI/ML to support FAIR data initiatives.
Train and Educate Employees: Provide training and education to help employees understand the importance of FAIR data and how to work with FAIR data effectively.
The Future of FAIR Data in Pharmaceutical R&D
The future of FAIR data in the pharmaceutical industry continues to offer hope for bringing new medicines faster to patients — particularly as the need for trusted and reproducible AI comes into focus.
As more organizations embrace and implement FAIR principles, we expect to see significant advancements in drug discovery, clinical trials, and regulatory compliance. By unlocking the power of their data, pharmaceutical companies can accelerate innovation, improve patient outcomes, and achieve sustainable growth.
Conclusion
The implementation of scaled and trusted AI, in conjunction with well-stewarded FAIR data will continue to transform the pharmaceutical industry…one R&D project at a time. By making data more findable, accessible, interoperable, and reusable, organizations will unlock new insights, accelerate drug development, and improve patient care. While challenges will arise, the benefits of FAIR data far outweigh the costs. By embracing FAIR data, the pharmaceutical industry will continue to lead in medical innovation and save lives.
Want to start a discussion and/or need help with your own FAIR data transformational journey? Contact me at — john.apathy@xponentl.ai
John Apathy
Chief Solutions Officer, Life Sciences
Tom Plasterer, Ph.D.
Managing Director, FAIR Data and Knowledge Graph Practice